Key Benefits Of Data Annotation
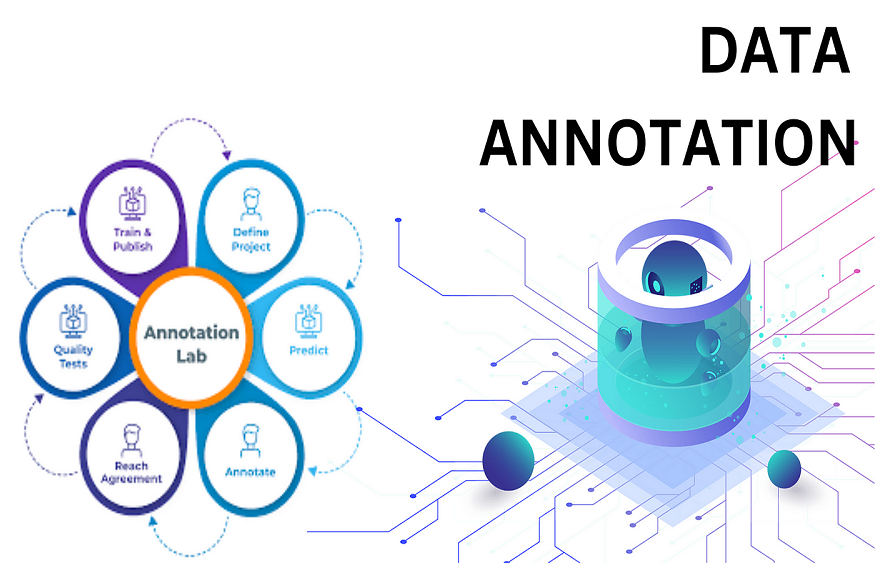
Artificial intelligence and machine learning are combinedly revolutionizing several industries globally. The utilization of machine learning and artificial intelligence have made applications wise, leading to workflow automation at every level of an organization. The global annotation market size was valued at 695.5 million USD in 2019, and this market size is expected to reach 6.45 billion USD by the end of 2027. The growth of artificial intelligence industries is driving the data annotation industry to witness magnificent growth with a CAGR of 32.54 per cent by 2027. Examples of industries that benefit from data labelling and annotation are autonomous vehicles, image and text annotation, and image or object recognition.
What is Data Annotation?
Data annotation can be defined as the process of data labelling present in several formats like images, videos, and texts. Data labelling helps identify objects with the help of computer vision and further trains the system with machine learning. This process includes the understanding and memorizing input patterns by the machine to create a dataset essential for machine learning. Different types of data annotation are practised in the industry, primarily in recognizing text, videos, and images as objects through computer vision.
Need for Data Annotation
Below are some customer pain points that make data annotation essential for several industries:
- Lack of quality control and quality assurance processes
- Poor monitoring
- Incapable software that was not designed for data labelling
Different Methods for Data Annotation
Different data annotation methods are available for industrial uses with a primary objective to help machines recognize text, videos, and images as objects by computer vision. The different types of data annotation methods are given below:
- Content and text categorization
- Bounding boxes
- Entity annotation
- Lines and Splines
- Images and video annotations
- Semantic segmentation
- Landmark and key point
- Polygonal segmentation
- 3D cuboid
Benefits of Data Annotation
Data annotation benefits from a reliable combination of artificial intelligence and machine learning.
Machine learning algorithms require labelled data to ensure learning and training. Here is why data annotation is effectively vital for data management services of organizations:
Flexibility
Machine learning has countless possibilities for perfect model performance, high-quality stability, and data security standards or a few essential measures in data annotation services. However, there are several other competitive parts like customer service and flexibility. The continuous development of machine learning models to enhance the performance eventually results in continuously changing workflow—this constant change in workflow results in variability and uncertainty in data labelling. The validation phase and model test require a quick and fast response to change workflow. As a result, labelling loop control and better engagement are critical competitive benefits that provide flexible solutions to organizations.
Accuracy
The machine learning assistance in data annotation eliminates human errors with automatic pre-labelling.
The real-time quality control and quality assurance are integrated into the annotation workflow as it has a consensus mechanism to improve accuracy.
The consensus mechanism includes indicating the answers referred by the majority.
The results of data annotation go through further assessment and verification that ensure up to 98% accuracy.
The machine needs to learn from new sets of data available to train. These datasets help train the machine for a productive output, with various data being used to train with different factors targetted to help the machine learning model. This dataset-based learning determines the accuracy of your data annotation model. The more you train, the more effective outcome you get.
Cost-effective communication
Several distributors offer several data labelling templates for quick labelling. Moreover, the collective contribution of the human workforce and machine learning helps reduce the cost by 50%.
Enhanced user-experience
Machine learning-based artificial intelligence applications offer a streamlined and seamless end-user experience. It is also being used in global search engines like Google to deliver effective and most appropriate results.
Self-control on data labelling
The data management service providers allow users to set labelling rules for better results. They offer a practical approach to manual labelling with a real-time estimation of price and time for data labelling.
How Can Outsourcing Data Annotation Help?
Outsourcing your data annotation is helpful as data annotation is a time taking and lengthy process. It requires manual overseeing of the ongoing process and thus requires a human workforce to administer the workflow. Outsourcing can help reduce the workforce burden on the organization with a practical and impactful data annotation. or labelling. Organizations like Apple and Lionbridge outsource their data annotation to extract these benefits.
Conclusion
Data annotation has become incredibly important for business and organizational workflows. This process refers to machine learning-based artificial intelligence models to help with data extraction from any text, image, or video. The need for data annotation exists as raw data or unlabelled data is useless for machine learning, and it cannot be processed with accuracy and sustained data loss. Data annotation is an essential part of data management for organizations undergoing extensive data processing. Several large-scale to small-scale organizations like Apple and Lionbridge globally utilize data annotation in their primary business workflow.